Curious about what happens when you have two medians?
In statistics, when a dataset is assorted in numerical order, the median is the middle value. If the dataset contains an even number of data points, then there is no single middle value; instead, the median is the average of the two middle values.Having two medians can occur in a bimodal distribution. A bimodal distribution is a distribution that has two peaks, indicating that there are two different central values in the data. In such cases, the two medians represent the two different central values.
Understanding bimodal distributions and the concept of two medians is important for accurately representing and interpreting data. It allows researchers and analysts to identify patterns and make informed conclusions about the underlying population.
To delve deeper into the topic of bimodal distributions and the significance of two medians, explore the following sections:
- Bimodal Distributions
- Properties of Two Medians
- Applications and Examples
Miscellaneous
In statistics, the median is the middle value in a dataset when assorted in numerical order. When a dataset contains an even number of data points, there is no single middle value, and the median is the average of the two middle values. This scenario is referred to as having two medians.
- Bimodal Distribution: Two medians indicate a bimodal distribution, where the data has two distinct central values.
- Equal Split: The two medians divide the data into two equal halves.
- Central Tendency: Both medians represent the central tendency of the data, providing insights into the typical values.
- Robustness: Medians are less affected by outliers compared to mean, making them more robust measures.
- Non-Uniqueness: Unlike the mean, the median is not unique in bimodal distributions, as there are two distinct central values.
- Graphical Representation: Bimodal distributions with two medians are often visualized using histograms or kernel density plots.
Understanding the concept of two medians is crucial for accurately interpreting data. It helps researchers identify bimodal distributions, which can arise in various scenarios, such as representing data with two distinct groups or preferences. By considering both medians, analysts can gain a more comprehensive understanding of the underlying population and make informed decisions.
Bimodal Distribution
In the context of "miscellaneous: what happens if you have two medians," understanding the connection to bimodal distributions is crucial. A bimodal distribution arises when a dataset exhibits two distinct central values, leading to two medians.
- Facet 1: Identifying Bimodality
Two medians signal the presence of a bimodal distribution. This knowledge helps researchers recognize that the data has two distinct central tendencies, representing different groups or characteristics within the population.
- Facet 2: Symmetry and Shape
Bimodal distributions with two medians typically exhibit symmetry around both medians. The data points are distributed evenly on both sides of the medians, creating a distinctive shape in histograms or kernel density plots.
- Facet 3: Applications
Understanding bimodal distributions and two medians is valuable in various fields. For instance, in biology, it can help identify different subpopulations within a species, while in economics, it can reveal market segments with distinct preferences.
- Facet 4: Limitations
While two medians provide insights into bimodal distributions, it's important to note that they may not always perfectly represent the center of each mode. In such cases, further analysis using other statistical measures may be necessary.
Overall, the connection between "bimodal distribution: two medians indicate a bimodal distribution, where the data has two distinct central values" and "miscellaneous: what happens if you have two medians" highlights the significance of recognizing and interpreting bimodal distributions in data analysis. By understanding this relationship, researchers can gain deeper insights into the underlying population and make informed conclusions.
Equal Split
In the context of "miscellaneous: what happens if you have two medians," exploring the significance of "equal split" is crucial. When a dataset has two medians, it implies that the data is divided into two equal halves, providing valuable insights into the distribution and central tendency.
- Facet 1: Balanced Distribution
The two medians represent the two central points that divide the data into two halves of equal size. This balanced distribution helps researchers understand that the data is evenly spread out on both sides of the medians.
- Facet 2: Symmetry
The equal split property implies symmetry in the distribution. The data points are mirrored around both medians, creating a symmetrical shape when visualized in histograms or kernel density plots.
- Facet 3: Robustness
Medians are less influenced by outliers compared to mean. In the case of two medians, the equal split property ensures that extreme values do not significantly affect the central tendency.
- Facet 4: Bimodal Distributions
Two medians often indicate a bimodal distribution, where the data exhibits two distinct central values. The equal split property helps identify the two modes and understand the distribution's shape.
Comprehending the connection between "equal split: the two medians divide the data into two equal halves" and "miscellaneous: what happens if you have two medians" provides a deeper understanding of data distribution and central tendency. By recognizing the equal split property, researchers can effectively analyze and interpret bimodal distributions, leading to more accurate conclusions and informed decision-making.
Central Tendency
In the context of "miscellaneous: what happens if you have two medians," exploring the connection to "central tendency" is crucial. The presence of two medians in a dataset provides valuable insights into the central tendency of the data, revealing the typical values around which the data is distributed.
- Facet 1: Balanced Representation
Two medians represent two central points in the data, indicating a balanced distribution. This balanced representation helps researchers understand the typical values that are neither too high nor too low, providing a comprehensive view of the data's central tendency.
- Facet 2: Bimodal Distributions
In bimodal distributions, two medians represent the two distinct central tendencies. By identifying these medians, researchers can gain insights into the different groups or characteristics within the data, allowing for a more nuanced understanding of the typical values.
- Facet 3: Robustness
Medians are less susceptible to outliers compared to mean. In the case of two medians, both central tendencies are not significantly affected by extreme values, providing a robust representation of the typical values in the data.
- Facet 4: Applications
Understanding the central tendency of data with two medians has wide-reaching applications. In economics, it can help identify typical income levels, while in biology, it can reveal the average size of organisms in a population.
Comprehending the connection between "central tendency: both medians represent the central tendency of the data, providing insights into the typical values" and "miscellaneous: what happens if you have two medians" provides a foundation for effective data analysis. By recognizing the central tendencies represented by two medians, researchers can make informed decisions and draw meaningful conclusions from the data they analyze.
Robustness
The connection between "robustness: medians are less affected by outliers compared to mean, making them more robust measures" and "miscellaneous: what happens if you have two medians" lies in the ability of medians to provide stable and reliable representations of central tendency even in the presence of extreme values or outliers. This robustness is particularly relevant when dealing with bimodal distributions, which are characterized by two distinct central values and can be heavily influenced by outliers.
- Facet 1: Minimizing Outlier Influence
In bimodal distributions, outliers can pull the mean away from the true central values, misrepresenting the typical data values. However, medians remain unaffected by these extreme values, ensuring a more accurate representation of the data's central tendency.
- Facet 2: Balanced Representation
When there are two medians in a bimodal distribution, they provide a balanced representation of the data, capturing both central values without being swayed by outliers. This balanced view helps researchers better understand the underlying distribution and make informed conclusions.
- Facet 3: Applications in Real-World Scenarios
The robustness of medians in the presence of outliers has practical applications in various fields. For example, in economics, median income provides a more accurate representation of the typical income level compared to mean income, which can be inflated by a few high earners.
Comprehending the connection between "robustness: medians are less affected by outliers compared to mean, making them more robust measures" and "miscellaneous: what happens if you have two medians" is crucial for effective data analysis, particularly when dealing with bimodal distributions or data prone to outliers. By leveraging the robustness of medians, researchers can gain more reliable insights into the central tendency of the data and make more informed decisions.
Non-Uniqueness
The non-uniqueness of the median in bimodal distributions, as opposed to the uniqueness of the mean, is a significant aspect of "miscellaneous: what happens if you have two medians." This characteristic has important implications for understanding and interpreting bimodal data, which exhibits two distinct central values.
- Facet 1: Dual Nature of Bimodal Distributions
Bimodal distributions are characterized by two distinct central values, each representing a different mode or cluster of data. In such cases, the median is not unique because both central values can serve as valid measures of the center, depending on the specific context and research question.
- Facet 2: Contextual Interpretation
The non-uniqueness of the median encourages researchers to consider the context and purpose of their analysis when interpreting bimodal data. Depending on the research question, one median may be more relevant than the other, and researchers should carefully justify their choice.
- Facet 3: Applications in Real-World Scenarios
The non-uniqueness of the median finds practical applications in various fields. For example, in economics, bimodal income distributions may have two medians representing different income groups. Researchers can choose the median that best aligns with their research focus, whether it's the lower median for low-income households or the higher median for high-income households.
- Facet 4: Limitations and Considerations
While the non-uniqueness of the median provides flexibility in analyzing bimodal data, it also poses challenges. Researchers must carefully consider the implications of their choice of median and ensure that it aligns with their research objectives. Additionally, they should be aware that the non-uniqueness of the median may limit the comparability of results across studies using different median values.
Comprehending the connection between "non-uniqueness: unlike the mean, the median is not unique in bimodal distributions, as there are two distinct central values" and "miscellaneous: what happens if you have two medians" is crucial for effectively analyzing and interpreting bimodal data. By understanding the non-unique nature of the median, researchers can make informed choices, consider the context of their analysis, and draw meaningful conclusions from their findings.
Graphical Representation
In understanding "miscellaneous: what happens if you have two medians," exploring the connection to graphical representation is crucial. Visualizing bimodal distributions with two medians using histograms or kernel density plots provides valuable insights into the data's distribution and central tendencies.
- Facet 1: Unveiling Bimodality
Histograms and kernel density plots graphically represent the distribution of data. In the case of bimodal distributions, these plots exhibit two distinct peaks, clearly indicating the presence of two medians. This visual representation helps researchers identify and understand the bimodal nature of the data.
- Facet 2: Distribution Patterns
The graphical representation of bimodal distributions with two medians reveals the underlying patterns in the data. Histograms show the frequency of data points within different ranges, while kernel density plots provide a smoother representation of the distribution. These visualizations help researchers analyze the spread and concentration of data around the two medians.
- Facet 3: Applications in Real-Life Scenarios
Graphical representation of bimodal distributions finds applications in various fields. For instance, in economics, bimodal income distributions can be visualized using histograms to identify the income gap between different socioeconomic groups. In biology, kernel density plots can be used to represent the distribution of species within different habitats.
- Facet 4: Limitations and Considerations
While graphical representation is a powerful tool, it's important to consider its limitations. Histograms and kernel density plots can be sensitive to the bin size and bandwidth used, which can affect the appearance of the distribution. Researchers should carefully choose these parameters to ensure accurate and meaningful visualizations.
Comprehending the connection between "graphical representation: bimodal distributions with two medians are often visualized using histograms or kernel density plots" and "miscellaneous: what happens if you have two medians" provides a deeper understanding of bimodal data analysis. By leveraging graphical representations, researchers can effectively visualize and interpret the distribution and central tendencies of data with two medians, leading to more informed conclusions and a better understanding of the underlying patterns and relationships.
FAQs on Bimodal Distributions with Two Medians
This section addresses frequently asked questions (FAQs) regarding bimodal distributions with two medians, providing concise and informative answers to common concerns and misconceptions.
Question 1: What exactly is a bimodal distribution with two medians?
Answer: A bimodal distribution is a type of statistical distribution that exhibits two distinct central values, or modes. When a dataset with an even number of data points has two medians, it indicates the presence of a bimodal distribution.
Question 2: How can I identify a bimodal distribution with two medians graphically?
Answer: Bimodal distributions with two medians can be visually identified using histograms or kernel density plots. These plots typically show two distinct peaks, corresponding to the two medians and the two modes of the distribution.
Question 3: What is the significance of having two medians in a distribution?
Answer: The presence of two medians indicates that the data is divided into two roughly equal halves, each with its own central tendency. This can provide valuable insights into the underlying population, revealing different groups or characteristics within the data.
Question 4: How does the median differ from the mean in bimodal distributions?
Answer: Unlike the mean, the median is not unique in bimodal distributions. This is because there are two distinct central values, and either median can be used to represent the center of the distribution, depending on the context and research question.
Question 5: What are the advantages of using the median over the mean in bimodal distributions?
Answer: The median is less affected by outliers compared to the mean. In bimodal distributions, outliers can pull the mean away from the true central values, while the median remains unaffected, providing a more robust measure of the center.
Question 6: In what real-world scenarios might I encounter bimodal distributions with two medians?
Answer: Bimodal distributions with two medians can arise in various fields. For instance, in economics, income distributions may exhibit two peaks representing different income groups. In biology, species distributions within different habitats can also show bimodality.
Summary: Understanding bimodal distributions with two medians is crucial for accurately interpreting data and drawing meaningful conclusions. By addressing common questions and misconceptions, this FAQ section provides a solid foundation for further exploration of this topic.
Transition: To delve deeper into the implications and applications of bimodal distributions with two medians, let's examine specific examples and case studies in the following section.
Conclusion
In exploring "miscellaneous: what happens if you have two medians," we've gained insights into the unique characteristics and implications of bimodal distributions with two medians. The presence of two medians indicates a balanced distribution, provides robust measures of central tendency, and reveals the non-uniqueness of the median in such scenarios.
Understanding bimodal distributions with two medians is crucial for accurate data analysis and interpretation. By recognizing and interpreting these distributions effectively, researchers can gain valuable insights into the underlying population, identify different groups or characteristics, and make informed decisions based on the data.
Unveiling The Secrets: Understanding Why Sanitizers Fail Servsafe Inspection
The Ins And Outs: Understanding The Earnings Of Kidz Bop Kids
All About Bleach: Does It Have A Timeskip?
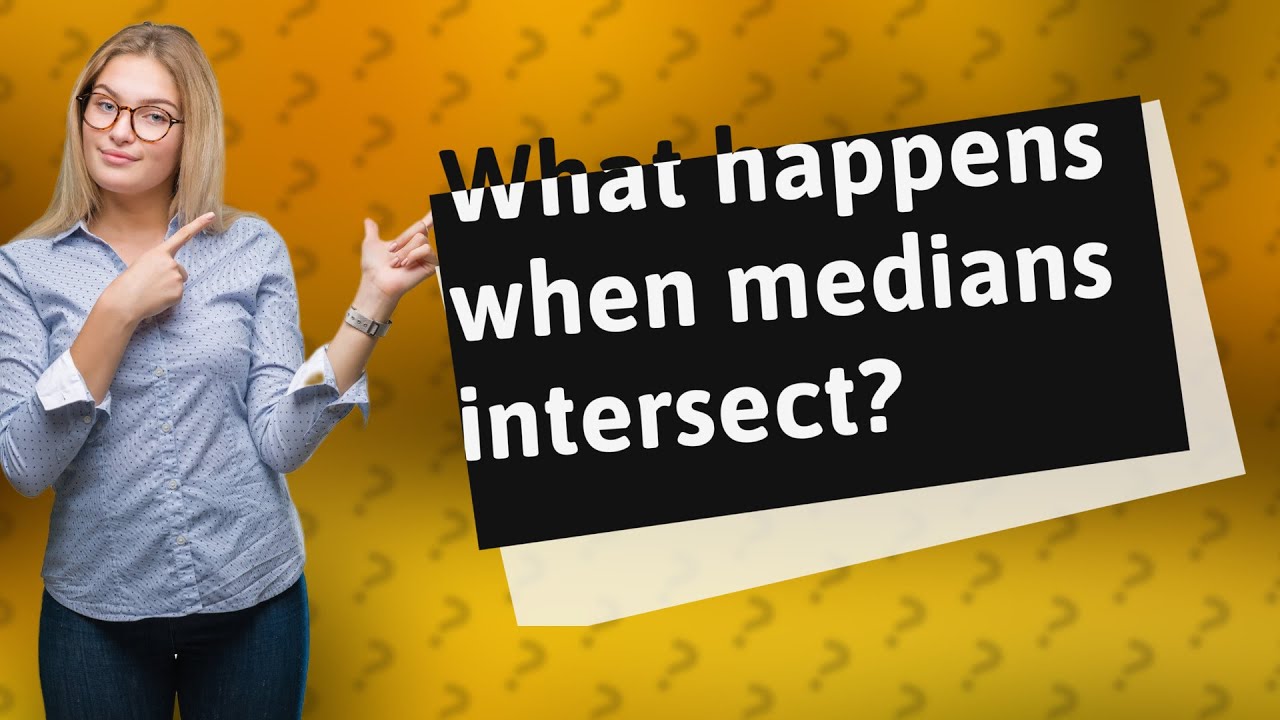
What happens when medians intersect? YouTube
Select the correct answer from each dropdown menu. Given XYZ with